Like it or not, Big Data is fast becoming critical to competing in the field of insurance from underwriting to rating in the property and casualty insurance markets. As a result, the National Association of Insurance Commissioners (NAIC) are taking up the gauntlet and their Innovation and Technology Task Force is focusing on offering consumer protections related to how that influx of data is utilized.
The deputy commissioner and chief actuary from the Alabama Department of Insurance, Charles Angell, says the mission of the Big Data (EX) Working Group is to help state regulators quantify what data is collected, how the data is collected and how insurers and third parties will use that information for marketing, rating, underwriting and claims-handling. Angell says the work of the group will identify concerns and benefits for consumers. It will also study such uses for compliance with state insurance statutes and regulations.
The NAIC says the Big Data (EX) Working Group will seek a clear understanding of what data insurers are collecting, how the data is being collected, and how it is used by insurers and third-party insurance operations.
So what is Big Data and how are companies using it?
Imagine a world where it’s suddenly possible to analyze such ephemera as “tweeting-while-drinking” patterns – or other alcohol-related behavior – and how it might effect insurer’s decisions.
Nabil Hossain and his team at the University of Rochester came up with an interesting idea.
They’ve trained a “machine” to identify alcohol-related tweets and demonstrated how that data can reveal alcohol-related behavior distributed throughout society. Aside from the fact that their methodology is capable of impacting society’s understanding and response to public health issues related to alcohol use and abuse, it’s certain to be employed to assess risk and actuarial practice in insurance markets.
It’s Hossain’s breakthroughs in machine-learning algorithms and “home locations” with great accuracy – down to identifying whether or not most drinking in a neighborhood takes place at home or in public venues – which will surely impact insurance decisions. By collecting Big Data in the form of geotagged tweets sent from New York City and from the city of Rochester, the analysts filtered tweets for mentions of alcohol or alcohol-related words. Words like “drunk,” “beer,” “party.” You get the drift.
The team then employed the power of Amazon’s Mechanical Turk crowd sourcing to analyze the tweets in fine detail. The Turk analysts then decided if a particular message referred to alcohol and, if so, whether or not the tweet revealed the message-sender was actually drinking alcohol. Drilling down further, the tweets were examined to determine if the sender was actually drinking at the very moment a message was sent. Using more than 11,000 geolocated tweets as a baseline, the data was used to “train” a machine-learning algorithm to spot alcohol-related Tweets.
As researchers are now capable of working out a user’s “home location” from a geolocated tweet and even identifying the place they most often message from, they time-coded the data. But that method isn’t granular enough to be entirely reliable. So the team dug deeper yet.
Hossain developed an approach which used common words and phrases like “Finally home!” or bath, sofa, TV and dinner to provide location keys.
Given that Hossain says he and his team can fix the site of a “home location” to within 100 meters (accurate to within 80 percent), the techniques essentially allowed the scientist to determine where and when people are drinking. By mapping liquor stores and bars in a given area, they found their sweet spot. The resulting “heat maps” not only reveal interesting patterns, they might one day be used to set insurance rates.
Imagine if you will an Insurech firm which sells “on-demand” and “metered” coverage. Data related to the density of alcohol outlets in a region – and even the number of tweets related to how many people are drinking in that region – will be used to set “premiums.”
Big Data applications such as Hossain’s are relatively inexpensive and speedy to employ when compared to a grind through reams of actuarial data, and they can be used in real-time.
No need to rely on inaccuracies inherent in analyzing data from questionnaires; machine-learning approaches could easily become the go-to tools for making actuarial decisions.
“Our results demonstrate that tweets can provide powerful and fine-grained cues of activities going on in cities,” says Hossain. “We can explore the social network of drinkers to find out how social interactions and peer pressure in social media influence the tendency to reference drinking,”
That’s where a close analysis of how Big Data becomes crucial to how such methodologies are used in fields and markets from insurance to finance. While data gathered from Twitter users would currently be heavily skewed toward younger people and over-represent them, other related data collection methods such as cell phone and internet history data will come into play as well.
As alcohol is the third-largest cause of preventable death in the U.S. – responsible for some 75,000 deaths every year – insurers can be expected to pay close attention to techniques like the work being done in Rochester.
To that end, the NAIC task force is considering whether or not “automation vendors” who develop statistical and analytic models such as Hossain’s should be examined like the insurance advisory organizations that develop “loss costs.” Task force members say consumers should be made aware of the extent to which data about them is being collected – and how that data is being applied to decisions related to the availability and cost of their insurance.
“How can consumers alter their risk characteristics if they don’t even know what data is collected. Should there be some kind of disclosure notice required?” Angell says.
Angell says he sees consulting teams examining such questions as “advisers” and not as “regulator.” Angell says he envisions such roles for groups being to inform insurance departments on “issues it found with the way a model is constructed.”
According to Angell determinations regarding compliance would remain with the states, and he says insurers will ultimately benefit from having technical objections to a proposal raised and addressed in a central forum.
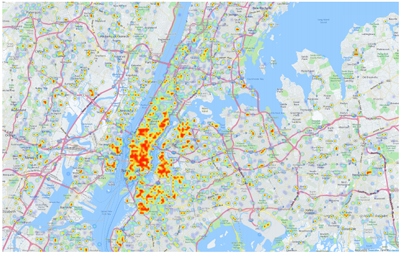